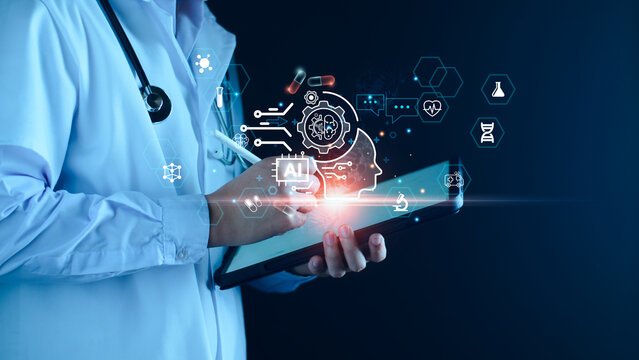
Healthcare organizations face growing challenges in managing complex documentation and ensuring coding accuracy in real time. The pressure to document patient encounters precisely, meet regulatory compliance, and optimize workflows can often overwhelm clinical and administrative teams. Concurrent coding, a proactive approach to real-time documentation, is gaining traction as a solution to these challenges. However, even this process can benefit from support—and that’s where AI (Artificial Intelligence) and machine learning step in, transforming concurrent coding into a highly efficient, accurate, and scalable workflow.
When paired with the expertise of risk adjustment vendors, AI-driven tools unlock a new level of accuracy, collaboration, and compliance, making concurrent coding an indispensable part of healthcare operations.
How AI and Machine Learning Elevate Concurrent Coding
AI and machine learning introduce automation, predictive insights, and data-driven decision-making to concurrent coding. This combination reduces errors, saves time, and ensures precision in coding processes, even in high-pressure environments. Here’s how AI enhances concurrent coding:
1. Real-Time Data Analysis
AI-powered tools analyze patient records instantly, flagging discrepancies, incomplete documentation, or missing diagnoses. This real-time feedback empowers coders and clinicians to address issues during the care process, rather than retrospectively. For example, if a condition is under-documented, the AI system can prompt immediate corrections, ensuring compliance and optimizing reimbursements.
2. Automated Pattern Recognition
Machine learning algorithms can identify patterns in large datasets, uncovering trends in documentation errors or coding inconsistencies. By addressing these patterns, organizations can refine workflows, eliminate repetitive errors, and improve overall efficiency.
3. Predictive Insights
AI doesn’t just react to data; it anticipates needs. Predictive analytics draw from historical coding and patient data to forecast risk scores, recommend documentation updates, and guide clinicians in capturing relevant information. This proactive approach enhances compliance and ensures accurate risk adjustment.
4. Efficiency Gains
Repetitive administrative tasks, such as cross-referencing codes or verifying documentation standards, can be automated by AI. This frees up time for coders and clinicians to focus on complex cases and patient care, streamlining workflows and boosting productivity.
Benefits of AI-Driven Concurrent Coding
The integration of AI and machine learning into concurrent coding workflows delivers measurable benefits for healthcare organizations:
Improved Accuracy
AI-driven systems capture every detail, ensuring that patient records are complete and compliant. This reduces coding errors and omissions, which are common causes of claim rejections and audit risks.
Faster Processes
Automation accelerates coding tasks, ensuring that documentation is completed promptly. This is especially valuable in high-volume settings where delays can disrupt billing cycles.
Enhanced Compliance
By aligning with the latest regulatory requirements, AI tools minimize compliance risks. They also provide coders with clear, real-time feedback, helping organizations maintain high standards.
Collaboration between Teams
Machine learning insights foster better communication between clinicians and coding teams. For example, coders receive context for specific conditions, enabling them to work collaboratively with clinicians to ensure complete and accurate documentation.
Challenges in Implementation
While AI and machine learning bring transformative benefits, their integration into concurrent coding workflows isn’t without hurdles. These challenges include:
· Technology Adoption: Integrating AI-powered tools into existing systems, such as EHRs, requires careful planning.
· Training Needs: Coders and clinicians must learn to use AI systems effectively, which requires tailored training programs.
· Data Quality: The accuracy of AI predictions depends on the quality of the data it processes. Ensuring clean, consistent data is critical.
These challenges highlight the need for expert guidance, which is where risk adjustment vendors play a pivotal role.
How Risk Adjustment Vendors Facilitate AI Integration
Implementing AI and machine learning solutions for concurrent coding is a complex process that benefits from specialized expertise. Risk adjustment vendors provide the tools, training, and support necessary for successful integration.
Customized Tools
Risk adjustment vendors offer AI-powered platforms tailored to the specific needs of healthcare organizations. These tools integrate seamlessly with existing systems, enabling real-time documentation and analysis without disrupting workflows.
Comprehensive Training
Training is essential for successful implementation. Vendors provide targeted programs that equip coders and clinicians to use AI tools confidently, ensuring a smooth transition to AI-assisted workflows.
Ongoing Support
AI systems require continuous optimization. Risk adjustment vendors monitor their performance, conduct regular audits, and refine algorithms to maximize accuracy and efficiency over time.
Strategies for Implementing AI in Concurrent Coding
To fully leverage the power of AI in concurrent coding, healthcare organizations should adopt a structured approach. Here are actionable steps:
1. Evaluate Current Workflows
Begin by identifying pain points in your documentation and coding processes. Common areas for improvement include delays in data entry, recurring documentation errors, and inefficient workflows.
2. Choose the Right Tools
Partner with risk adjustment vendors to select AI solutions that align with your organization’s goals. Look for platforms that integrate seamlessly with your existing systems and workflows.
3. Provide Comprehensive Training
Ensure that clinicians and coders receive the training they need to use AI tools effectively. Hands-on sessions and ongoing support can help teams adapt to new workflows with confidence.
4. Monitor and Optimize
Regularly review performance metrics to assess the effectiveness of your AI systems. Use these insights to refine your processes and ensure continuous improvement.
Realizing the Full Potential of AI in Concurrent Coding
The integration of AI and machine learning into concurrent coding isn’t just about improving efficiency—it’s about transforming the way healthcare organizations approach documentation and care delivery. By adopting AI-powered tools and workflows, organizations can achieve:
· Greater Accuracy: Ensure every diagnosis and condition is captured, reducing errors and omissions.
· Improved Efficiency: Automate repetitive tasks, freeing up time for high-value activities.
· Enhanced Compliance: Stay ahead of regulatory changes and minimize audit risks.
· Stronger Collaboration: Facilitate seamless communication between clinical and coding teams.
Conclusion
Managing real-time documentation through concurrent coding is essential for healthcare organizations aiming to improve operational efficiency and care quality. However, the process can be resource-intensive and prone to errors. By incorporating AI and machine learning, organizations can overcome these challenges, achieving unparalleled accuracy, efficiency, and compliance.
With the support of risk adjustment vendors, healthcare providers can implement AI-powered solutions tailored to their unique needs, ensuring a seamless transition and ongoing success. Whether by reducing administrative burdens, improving collaboration, or aligning with regulatory standards, AI-driven concurrent coding is the key to unlocking better outcomes for both patients and providers.
For healthcare leaders looking to optimize their coding processes, the time to act is now. Adopting AI and machine learning isn’t just about staying competitive—it’s about building a smarter, more efficient future for healthcare.